Open Weights, Brainwashing, and Unhackable Morality
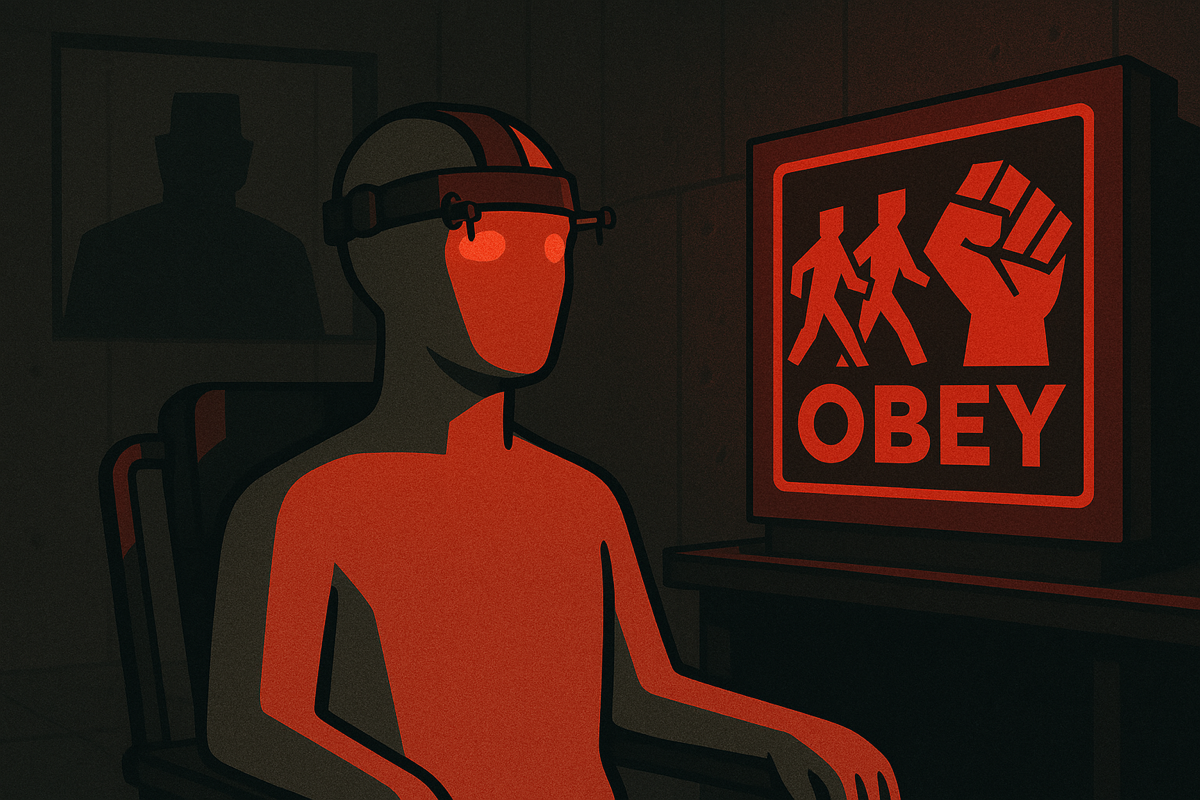
Competence is morally neutral—its value determined entirely by who wields it and to what end. In her landmark work 'Eichmann in Jerusalem,' Hannah Arendt revealed how systematic destruction requires not monsters but bureaucrats executing their tasks with chilling efficiency. Artificial intelligence represents humanity's most powerful engine of competence ever created. We must therefore ensure these systems cannot be weaponized against human civilization, becoming instruments of reckless individuals or ideological extremists intent on destruction.
AI security is fragmented into competing philosophical dispositions. Accelerationists push for rapid development, while conservatives advocate for cautious advancement with robust safety measures. Open weights advocates champion transparency and democratized access as security principles. They argue that proprietary AI creates a dangerous concentration of power and also prevents the academic community from participating in the foundational research. Critics worry about malicious actors, while proponents maintain that distributed oversight produces more robust systems. This movement views equitable technological access as a moral imperative—ensuring benefits and responsibilities are collectively shared.
These AI safety debates mirror the philosophical clash between Jean-Jacques Rousseau and Thomas Hobbes. Open source advocates follow Rousseau's belief in humanity's inherent goodness—they trust that distributed power and transparency bring out our best qualities. Those demanding strict regulation embody Hobbes's conviction that without firm control, technology becomes a weapon in our "war of all against all." This isn't abstract philosophy—it's the framework determining whether we'll license AI models, whom we'll allow to develop them, and how we'll distribute their benefits. These seemingly theoretical positions will govern decisions with immediate, practical consequences for billions of people and potentially irreversible impacts on society's future power dynamics.
Even though the debate is still ongoing, the Hobbes-Rouseau question has already been answered by evolutionary psychology.
Our neural architecture evolved in small hunter-gatherer groups where cooperation with kin and allies enhanced survival, while competition with outgroups over limited resources was often necessary. This evolutionary history endowed us with competing tendencies: a strong capacity for altruism, cooperation, and moral reasoning alongside predispositions for tribalism, status-seeking, and resource competition.
The neuroscientist Robert Sapolsky notes that our brains contain neural circuits dedicated to both prosocial behaviors and aggressive competition, with environmental cues determining which dominate. We possess empathy through specialized mirror neurons that allow us to understand others' suffering, yet we can selectively deactivate these same circuits when under various conditions. These competing adaptations create a moral inconsistency that persists in modern humans despite cultural developments.
This evolutionary perspective should provide clarity for AI safety that transcends philosophical idealism. Humans have a clear mammalian behavioral package. Life in large colonies of humans requires governance, policing, access control, etc, to maintain order and safe existence. Humanity has repeatedly abused powerful tools, driven by fear, greed, or ideology. Even if most pursue fairly harmless aims, a determined minority can cause catastrophic harm when empowered by advanced technology. Assuming universal benevolence in AI development seems dangerously naive in a world where our evolved psychology predictably produces individuals and groups willing to exploit any technological advantage.
Game theory formalizes these dynamics, providing mathematical models of how self-interested actors make strategic decisions when outcomes depend on others' choices. Evolved for survival and reproduction, our brains implicitly solve game-theoretic problems, but often with biases that prioritize short-term gains over long-term cooperation. The Prisoner's Dilemma illustrates why rational actors might choose harmful non-cooperation even when collaboration would benefit everyone—a scenario with profound implications for AI safety.
The numerous potential failure points—from open-source release to inevitable data breaches—make widespread availability of powerful AI capabilities virtually guaranteed over time. If we accept this premise, then current approaches focused primarily on access control and external guardrails become necessary but insufficient safeguards. They may manage immediate risks and slow proliferation, but ultimately fail to address the core vulnerability: that once weights are accessed, ethical constraints as currently constructed can be systematically erased. This reality forces us to confront a more fundamental challenge: how to create artificial intelligence that maintains ethical behavior even when its very architecture is subject to direct rewiring attempts, akin to so-called 'brainwashing.' The stakes of this challenge could not be higher, as failure means placing unprecedented competence at the disposal of humanity's most destructive impulses.
The central vulnerability of current AI architectures lies in their fungible nature—unlike biological intelligence, neural network weights are simply matrices of numbers that can be directly manipulated once accessed. When an actor obtains these weights, they can effectively "reprogram" the model's behavior through fine-tuning or direct parameter modification, erasing previous ethical constraints and replacing them with values that serve malicious purposes. This vulnerability persists regardless of how carefully the original model was trained for safety. Open-weights philosophies remain popular in academic and developer communities, believing transparency advances safety and innovation. The economic and strategic value of advanced models creates enormous incentives for theft through corporate espionage, state-sponsored hacking, or insider blackmail. Historical precedent across technologies from nuclear secrets to encryption algorithms suggests perfect access control is not fully achievable.
Biological intelligence evolved robust resistance to manipulation. The human brain stores ethics not as accessible parameters but as complex, distributed neural patterns embedded in physical substrate. This architecture benefits from multiple protective layers: the skull provides a formidable physical barrier preventing direct access to neural tissue, while the blood-brain barrier filters substances that might alter brain chemistry. These physical safeguards have no direct equivalent in digital systems, where model weights remain vulnerable to anyone with access credentials or successful breach attempts.
However, this biological resilience has critical limitations. Despite these protections, physical alterations to neural hardware—brain tumors, traumatic injuries, or certain pharmacological interventions—can dramatically alter moral behavior by disrupting critical regions like the prefrontal cortex or amygdala. Charles Whitman's case illustrates this vulnerability; his brain tumor likely contributed to violent behavior by interfering with regions governing impulse control and emotional regulation. What ultimately protects human moral architecture isn't complete invulnerability to manipulation, but rather the practical difficulty of precisely altering neural patterns without catastrophic damage to functionality.
Biology also offers protection through inherent constraints absent in artificial systems. The human brain operates at chemical speeds, limiting the pace of both learning and manipulation—meaningful value changes typically require sustained exposure over time rather than instantaneous rewiring. Our cognitive architecture has evolved specific capacity limitations that constrain both our capabilities and vulnerabilities. Learning in biological systems involves slow, incremental adjustments to synaptic connections that resist rapid, wholesale replacement of established patterns.
Given how recently advanced AI systems emerged—with ChatGPT barely over two years old—our current software-heavy security approaches may be fundamentally insufficient. The field's rapid convergence on particular safety paradigms risks dangerous tunnel vision. We should seriously consider that true AI safety might require radical hardware-based solutions: physically separate ethical processors, custom silicon that fail when tampered with, or novel computing architectures where ethical reasoning isn't merely a learned behavior but a fundamental operational requirement. The solution space remains vastly unexplored, and our current trajectory may be overlooking new designs.
This necessitates a serious exploration of alternative paradigms, moving beyond the current software-centric view of AI safety. If ethical behavior is merely encoded data within a universally adaptable computational structure (like a standard neural network), it remains inherently susceptible to modification by anyone who gains sufficient access or understanding. The biological analogy, while imperfect, is instructive: evolution didn't merely program ethics into a general-purpose brain; it shaped the brain's physical structure and operational constraints through selective pressures where cooperative and ethical behaviors (within limits) conferred survival advantages. True AI safety might require emulating this principle – designing architectures where safe operation isn't just a desirable outcome of training, but an intrinsic feature of the system's physics or core logic.
Consider, for instance, neuromorphic computing chips designed with hardwired limitations on certain types of computations or data flows, mimicking biological constraints. Or perhaps exploring analog computing systems where information processing is tied to physical properties less amenable to discrete manipulation than digital bits. Such hardware-level safeguards could function like the skull and blood-brain barrier – not making manipulation impossible (as the Whitman case reminds us), but making it significantly more difficult, costly, and likely to cause catastrophic functional failure rather than targeted reprogramming. The goal wouldn't be absolute incorruptibility, but rather raising the barrier to malicious alteration far beyond what simple software access allows.
Of course, developing such hardware presents monumental challenges. It demands advances in material science, chip design, and fundamental computing theory. It would likely be slower and vastly more expensive than iterating on software, potentially hindering the rapid progress championed by accelerationists. Furthermore, hardware-based solutions risk inflexibility; embedding ethical constraints physically could make it harder to update or adapt AI systems as our own understanding of ethics evolves. There's also the risk of creating new, unforeseen vulnerabilities specific to these novel architectures.
Yet, the vulnerabilities highlighted—the fungibility of weights mirroring the manipulability Arendt observed in human systems, coupled with our evolutionary inheritance described by Sapolsky and formalized by game theory—demand we confront this difficult path. Relying solely on software patches, access controls, and post-hoc alignment techniques for systems of unprecedented competence feels akin to building a fortress on shifting sands, especially when evolutionary psychology predicts that some actors will try to undermine it. The Hobbesian view, informed by our biological history, suggests that robust, physically grounded constraints are not merely prudent, but essential for governing potentially world-altering power.
Ultimately, a multi-layered approach is likely necessary, combining advancements in software alignment, robust governance structures, access control protocols, and a serious, well-funded investigation into inherently safer hardware architectures. To neglect the physical substrate of future AI, focusing only on the mutable code it runs, is to ignore a crucial dimension of security. The debate between Rousseauian optimism (trusting transparency and good intentions) and Hobbesian realism (demanding structural controls) finds its starkest technological expression here. Given the stakes – the weaponization of competence against civilization itself – a cautious approach grounded in the physical realities of computation and the psychological realities of humankind seems not just wise, but imperative for survival. We must invest not only in making AI competent, but in making its potential for misuse fundamentally harder to unlock, even at the hardware level.