The Big Opportunity in AI: Legacy Industry
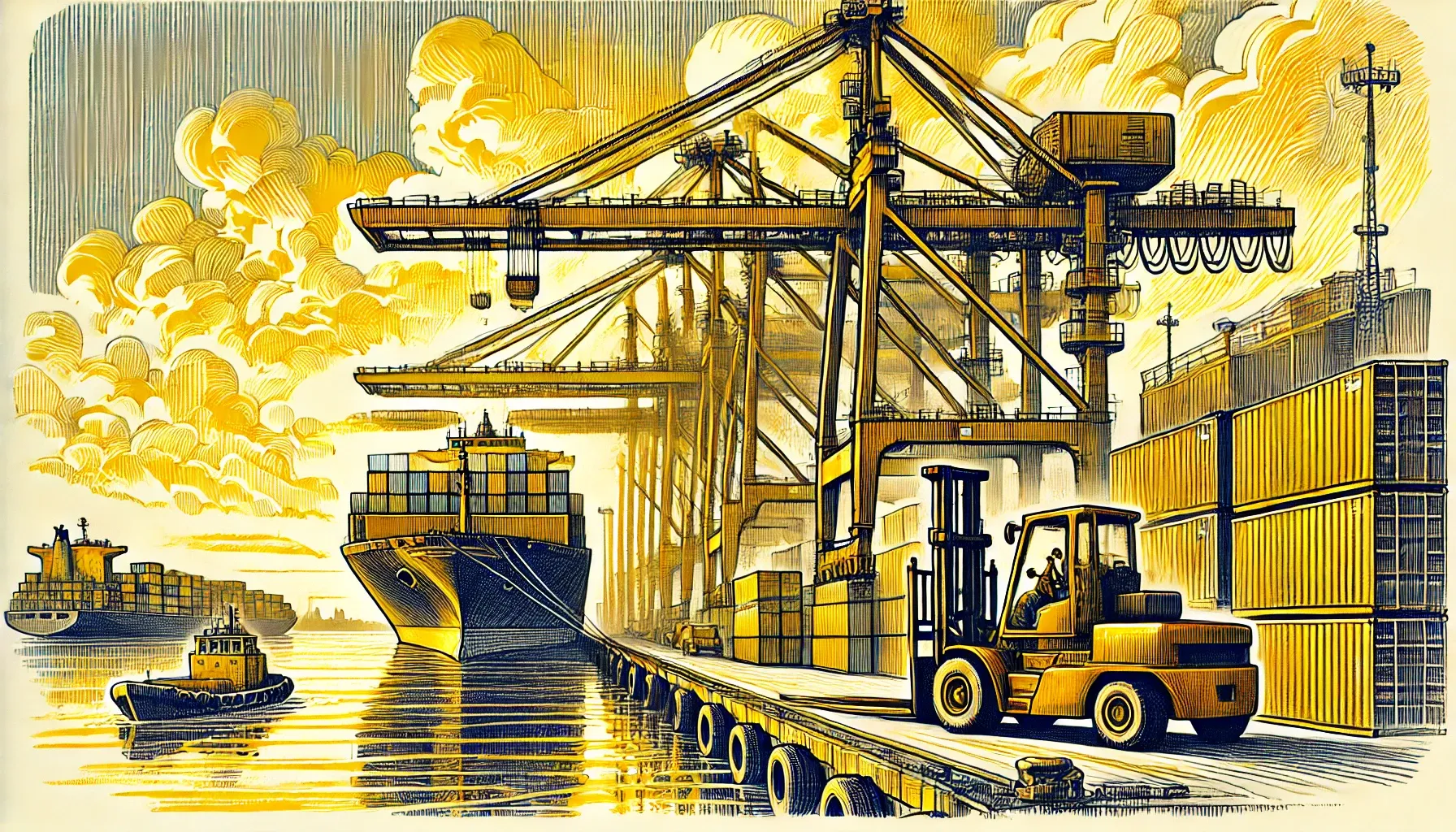
Over the past two years, artificial intelligence has become the centerpiece of a modern gold rush. Venture capitalists, corporate R&D departments, and national governments are pouring resources into AI development, each chasing the promise of the next breakthrough. The frenzy feels almost inevitable—a product of the outsized ambitions and anxieties stirred by an emerging technology that seems capable of redefining everything.
Yet beneath this frenzy, what you see is not a free-for-all but a narrowing of the field. The largest players—Google, OpenAI, Anthropic, xAI, Meta—are consolidating their lead in foundational AI research, building systems so large and costly that most competitors will never hope to match them. Startups, caught in the excitement, are trying to carve out their piece of this market by adding marginal improvements, niche applications, or features atop these systems.
But while attention remains locked on the AI infrastructure race, a far more profound transformation is beginning. The foundation models that seemed like science fiction just months ago are now reality. The real story isn't about who will build them—that question is largely settled. It's about how these technologies will transform the entire legacy economy. Every industry that hasn't yet been remade by software is now vulnerable to disruption. For entrepreneurs, the opportunity isn't in trying to compete in an already-decided infrastructure race, but in wielding these new tools to reshape traditional industries.
History shows us how easy it is to miss transformations by focusing on the wrong race. During the American railroad boom, public attention fixated on laying track—yet the greatest fortunes were built by those who saw past the infrastructure to how rail would reshape commerce. Standard Oil mastered logistics, Swift revolutionized meat distribution, and Sears reinvented retail. The same pattern emerged in the internet era. While the spotlight remained on infrastructure plays, Amazon quietly began dismantling traditional retail, Netflix transformed entertainment, and Square revolutionized payments. In each case, the lasting opportunities weren't in building the technology but in seeing how it would remake traditional industries.
This pattern is not coincidental. Clayton Christensen's research on disruptive innovation explains why these moments of technological change so often favor new entrants over incumbents. Through extensive study of industries from steel to storage, Christensen showed how success itself becomes a barrier to adaptation. Established companies excel at what he termed "sustaining innovations"—improvements that serve existing customers better. But they systematically fail at disruptive innovations that start as seemingly inferior solutions before rapidly reshaping entire industries. The very capabilities that make companies successful in stable times become handicaps during periods of transformation.
Consider how this played out during the internet revolution. Borders responded to Amazon by outsourcing its online operations to Amazon itself. Blockbuster rejected multiple opportunities to acquire Netflix, viewing streaming as inferior to its retail experience. Walmart, despite massive resources, took decades to build meaningful e-commerce capabilities. In each case, incumbent organizations couldn't embrace solutions that threatened their existing operations, relationships, and revenue models.
This dynamic is particularly clear in freight logistics, which moves $9.1 trillion of goods annually yet operates on systems that would be familiar to workers from fifty years ago. Traditional freight brokers still coordinate shipments through a byzantine network of phone calls, emails, and manual processes. The result is staggering inefficiency: twenty percent of trucks run empty at any given time, while goods sit idle waiting for coordination between siloed systems. The environmental cost is equally severe—empty miles alone generate an estimated 100 million metric tons of unnecessary carbon emissions annually.
An AI-native logistics business could reimagine the entire flow of goods. Machine learning models could optimize not just individual routes but entire networks, predicting demand patterns and dynamically adjusting to disruptions. Real-time pricing algorithms could eliminate costly negotiations, while automated systems handle documentation and compliance. Early implementations suggest cost reductions of forty to sixty percent. Traditional players understand this potential but face nearly insurmountable barriers to adoption. Their customers, trained on high-touch service models, resist automation. Their employees, compensated for relationship management, fight changes that would eliminate their roles. Their systems, built for human decision-making, require complete replacement.
The insurance industry presents an equally profound opportunity. Traditional insurers collect $5.5 trillion in annual premiums globally yet operate on actuarial models and organizational structures that haven't fundamentally changed since the early 20th century. Claims processing averages thirty days, with vast bureaucracies performing manual underwriting and assessment. The human factor creates both inefficiency and inconsistency—similar claims receive different treatment based on individual adjusters, while fraud detection relies heavily on intuition and spot checks.
An AI-native insurance company could reconstruct the entire stack. Machine learning models could assess risk dynamically using real-time data from connected devices, satellites, and other sources. Natural language processing could automate claims intake and processing, while computer vision systems could assess damage from photos or videos. Smart contracts could handle straightforward claims automatically, with human experts focusing only on complex edge cases. Early experiments in auto insurance show the potential: processing times reduced from weeks to minutes, fraud detection rates improved by sixty percent, and operating costs cut by more than half. Similar opportunities exist across property, life, and commercial insurance lines, where traditional carriers remain bound by manual processes and outdated risk models.
This opportunity extends far beyond the developed world. In fact, emerging markets may prove the most fertile ground for AI-driven disruption. Countries across Africa, Southeast Asia, and Latin America face a unique circumstance: large populations underserved by traditional industries but increasingly connected through mobile technology. These markets can potentially leapfrog legacy systems entirely, just as they bypassed landlines for mobile phones and branch banking for digital payments.
For entrepreneurs willing to look beyond Silicon Valley, these markets offer something precious: the chance to build without constraints. While developed economies navigate complex regulations and entrenched interests, emerging markets present a clean slate. A traditional US hospital has decades of established procedures, existing software systems, and organizational structures that resist fundamental change. An entrepreneur building a new healthcare delivery system in Rwanda can start fresh, designing AI-first systems that wouldn't be possible in markets with more entrenched incumbents.
The trajectory is becoming clear: as these technologies mature, they will remake sector after sector of the legacy economy. Traditional businesses, built around human cognitive capabilities, will face competition from AI-native companies operating with fundamentally different economics. While the giants of AI focus on improving foundation models, the sharpest entrepreneurs will see past the infrastructure race to the bigger opportunity: wielding these tools to manifest a whole new economy.